Churn Prediction - Maximize Your Customer Retention
A 5% increase in customer retention produces more than a 25% increase in profit. In the past, there were lots of business articles and research describing the customer retention and all of them came to the same conclusion: It is cheaper to keep existing customers than gain new ones. Over the years, we have collected a lot of experience with churn prediction, from industries like telecommunication providers, banking or computer security. Today we would like to share some of our experience with you.
The Underlying PROBLEM:
The main goal is to improve customer retention. The churn rate is the percentage of customers who discontinue their subscriptions to the service within a given time period. For a company to expand its customer base, its growth rate, as measured by the number of new customers, must exceed its churn rate. Furthermore, it is crucial to have a churn rate as low as possible to achieve a healthy growth.
Two CHALLENGES:
There are a couple of challenges in churn prediction. Mainly, there is no easy generic approach that will work for everyone. Something else works for each customer... for example, different types of discounts, products or simply, a more personalised company approach.
The second problem is that churners can't be identified too late, when they are already upset, or when they already know about good offers from the competition. Once a customer decides to take another offer, it is very hard to convince them to change their mind.
The SOLUTION:
Most of the companies turn to Machine Learning to solve this problem. There is more than one way to approach the problem.
The first way is to create a metric of the behavior of the customers, for example, the number of off-net calls in the last 30 days or how many times a customer visited the website of a competition in the last 10 days. Using features like these, we can create a Machine Learning model that predicts the probability of churn for each of our customers.
The second approach is the so-called “path to churn”. We can generate a number of events which follow a customer throughout their journey. Then we look for paths (sequence of events) which often lead to churn. Using this approach we can also predict what type of event will happen next for each customer.
The third approach is through influencing customers. The goal is to identify people, so-called Alpha Users or Influencers, who have a significant impact on the people in their network.
In A Business case:
Suppose we have a company with 1000 customers and a 5% churn rate. Using following assumptions we can compute the value of the churn prediction model.
Every month the company loses 50 customers
With their marketing budget the company can target 100 people
If they don't use Machine Learning to select people for their campaigns, they will target 100 people who will have 5 churners among them. Based on our experience, the lift of churn models can easily reach approximately 4 for the first decile of the customer base. That means when we target 100 people using a churn model there will be 20 churners among them. Using Machine Learning, the company will be able to keep more clients using the same campaign.
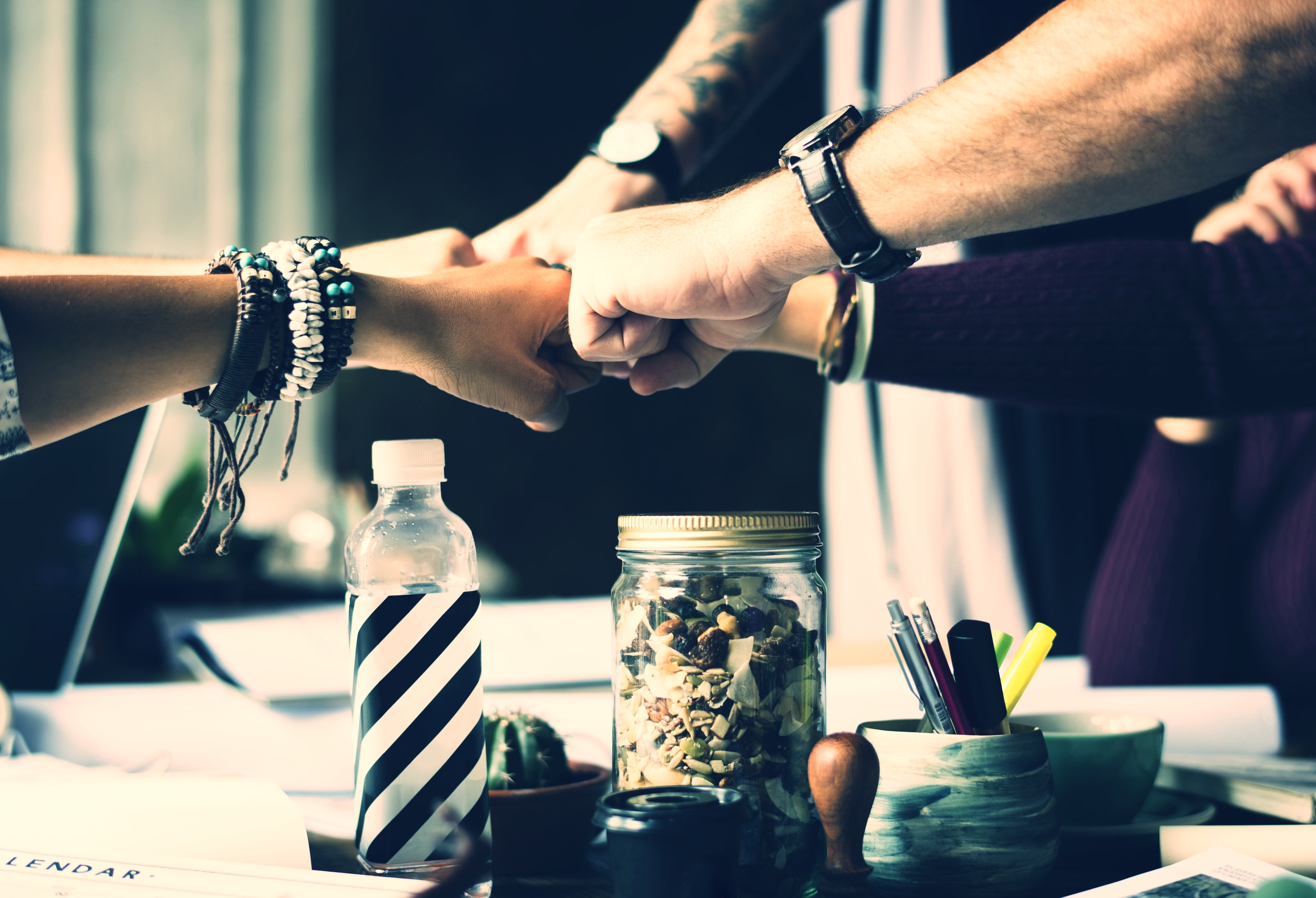
Our Value proposition:
The biggest value consists of the increase in revenue and the improvement in the targeting of campaigns. If you send discounts to people who would stay anyway, you will use revenue, but if you send discounts to the right people and make them stay, your revenue will increase. If you are currently thinking about tackling a similar problem in your business, don't hesitate to contact us.